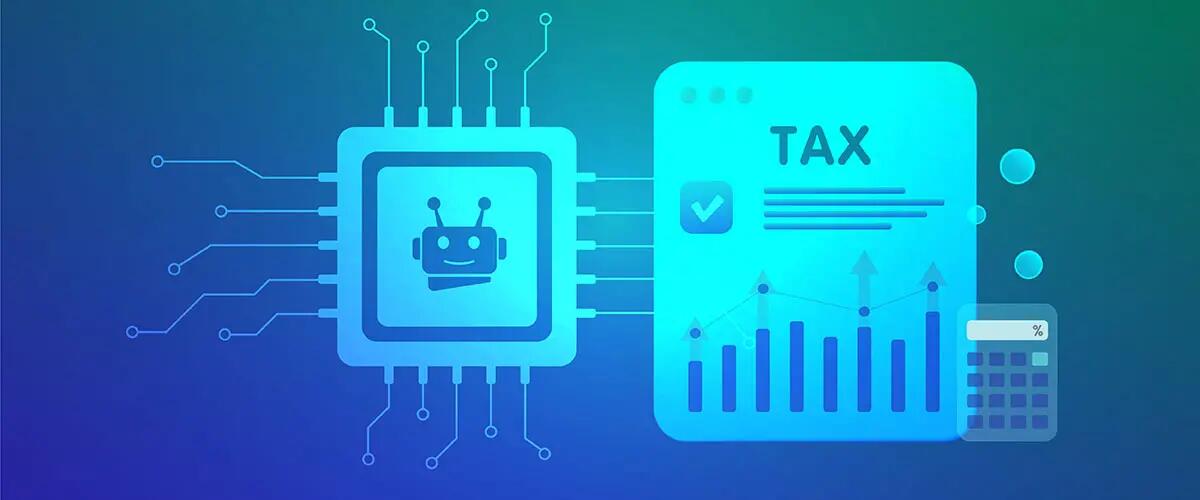
Getty Images
Thought Leadership: Machine Learning and the Future of Tax Valuation
This article, written by Rutgers Business School professor Jay A. Soled and North Carolina School of Law professor Kathleen DeLaney Thomas, was originally published on Columbia Law School's Blog on Corporations and the Capital Markets, May 20, 2022.
The tax law handles many computational issues with extraordinary efficiency. Need to compute employment taxes? The Internal Revenue Code (“Code”) lays out the rate for doing so. Need to ascertain the dollar amount deductible for business mileage expenses? Treasury regulations provide a formula based upon the number of miles driven.
But the same computational efficiencies do not routinely extend to asset valuations. As we discuss in in a recent article, administration of the tax law in this realm is plagued with problems. Admittedly, the problem is not universal; for example, it does not extend to (i) items that are bought or sold in an arm’s-length transaction, such as inventory, or (ii) assets that are traded regularly on a public exchange, such as marketable securities. Yet, assets not falling within either of those categories are ordinarily difficult to value and provide a fertile platform for taxpayers to strategically minimize their taxes.
Consider two examples. In the first, a taxpayer wishes to make a charitable contribution of a one-acre parcel of land. She secures the following three valuations for the land, each from a different appraiser: $200,000, $250,000, and $300,000. Assuming the taxpayer’s effective tax rate is 40 percent, were she to report the highest value, she would save $40,000 in income tax relative to if she had chosen the lowest value. Alternatively, suppose the same taxpayer wishes to gift the property to her daughter. Rather than the highest asset valuation appraisal, she may choose to use the lowest one, potentially saving her $40,000 of gift tax.
There are several reasons for the prevalence of asset valuation problems. First, there is information asymmetry between taxpayers and the IRS, with taxpayers having more information than the IRS, meaning they can camouflage an asset’s true value. Second, there are few incentives for taxpayers to report conservative valuations In the worst-case scenario where a taxpayer is actually audited, she bears little to no risk of being penalized, due to the inherent range of possible asset values. And since many courts are known for splitting the taxpayer’s and IRS’ valuation differences, it makes sense for taxpayers to take aggressive tax reporting positions as a starting point. Third, the judges themselves often have little or no valuation expertise and hence are at the whim of feuding parties and their parade of appraisal experts. Finally, many professional appraisers, in producing their work product, use a broad range of methodologies, none of which the Code sanctions.
Despite these problems, machine learning has been transformative in making asset valuations more reliable and efficient. Evidence for this proposition abounds. Most people are familiar with websites such as Zillow that, with a few keystrokes, provide instantaneous real estate appraisals. In the last few years, however, machine learning’s ability has been greatly augmented, going well beyond real estate, providing accurate appraisals for artwork, closely-held business interests, and a host of other assets as well.
Given the success of machine learning in asset valuation, Congress should consider reforming the Code and, when it comes to asset valuations, requiring the use of machine learning. In our article, we envision the following three steps:
- Step 1: Treasury taps into industry-developed machine-learning platforms such as Zillow to value assets or, alternatively, it develops its own asset valuation platforms.
- Step 2: Treasury implements the use of machine-learning valuation incrementally, starting with real estate.
- Step 3: Machine-learning asset valuations would be deemed to be presumptively correct; however, taxpayers could choose a different asset valuation utilizing a disclosure statement.
Were Congress to institute this three-step process, the effects would be twofold.
For starters, the administrative efficiencies would be immense. The time, effort, and resources taxpayers devote to securing asset appraisals would be greatly diminished. And since machine-learning valuations would be presumptively correct, the incentive to take overly aggressive valuation positions (in the hopes a court would split the difference in a dispute) would dissipate. By the same token, the IRS would have a valuation tool in hand that could readily crosscheck a taxpayer’s asset valuation. The courts, too, would be far less burdened by having fewer valuation cases on their already busy dockets.
Second, relative to other suggested methods to value assets, a machine-learning approach could be easily instituted. To curb valuation abuses, some commentators, for example, have recommended that if a taxpayer uses a particular value for tax purposes, the IRS would then have the right to purchase such property at that price and sell it at a public auction. A machine-learning approach to valuation would be far less intrusive than this or other proposed valuation methods.
The tax law should capitalize upon all new technologies to ensure taxpayer compliance and facilitate the tax administration process. An excellent place to start would be to employ machine learning to determine asset valuations. Doing so could alleviate taxpayers, the IRS, and courts of nettlesome valuation issues. At the same time, it would open the door for other reforms that rely on accurate asset valuation, such as the introduction of a wealth tax.
This post comes to us from Jay A. Soled, a professor at Rutgers Business School and director of its Masters of Taxation Program, and Kathleen DeLaney Thomas, the George R. Ward Term Professor of Law at the University of North Carolina School of Law. It is based on their recent article, “AI, Taxation, and Valuation,” forthcoming in the Iowa Law Review and available here.
Press: For all media inquiries see our Media Kit